Seeing the Difference a Dollar Makes
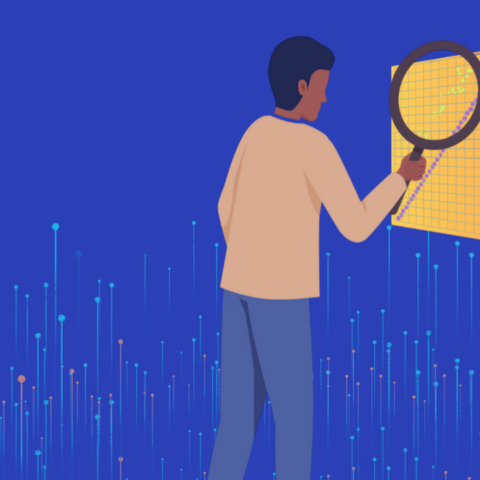
Most people can count cash. But measuring its effects isn’t so easy.
As a labor economist in the Jeb E. Brooks School of Public Policy, Zhuan Pei studies the efficacy of social and employment programs such as Medicaid, cash welfare, and disability insurance. Enrollees in these programs are often from underresourced communities. “We aim to rigorously evaluate whether these programs work or not,” he says. “What is their effect? What about them is most helpful to the people who participate in them?”
To answer these questions, Pei must apply statistical methods that he can trust to deliver accurate insights from staggering amounts of data. When expressed mathematically, these statistical methods look like a fierce breed of multivariable algebraic equations. Representing the same methods as graphs, however, tames them somewhat. “I spend a lot of my time looking at graphs,” Pei says. “We rely on them quite a bit to convey our findings. It helps to be able to express our work in ways that people can visually understand.”
Two methods that have captured Pei’s imagination are the regression kink design and regression discontinuity design, and they are notable for their use of graphs to convey empirical results.
The popularity of these methods among economists in recent years can be attributed in part to Pei himself. In addition to being a labor economist, Pei is an applied econometrician who develops and refines statistical methods to better understand the effects of policies. In a series of studies, Pei has investigated regression kink design and regression discontinuity design, exploring their value for finding causal relationships in complex data sets.
Regression Kink Design
While a doctoral student at Princeton, Pei was invited in 2009 to join a regression kink design project. Among his collaborators was labor economist David Card (University of California, Berkeley). (Card would go on to share the 2021 Nobel Memorial Prize in Economic Sciences with two other scholars, Joshua Angrist and Guido Imbens. In announcing the award, the Nobel Prize noted, “This year’s Laureates . . . have provided us with new insights about the labour market and shown what conclusions about cause and effect can be drawn from natural experiments.”)
The project with Card generated a seminal paper on the theory and practice of regression kink design. Coauthored by Card, David S. Lee, Pei, and Andrea Weber, the paper is regularly cited by economists and taught in graduate courses around the world.
“The idea behind regression kink design dates all the way back to the 1970s,” Pei says. “Some brilliant people came up with it, but they didn’t coin the name, and the model they proposed was a little restrictive. The work that I was part of at Princeton helped formalize a modern foundation for its widespread application.”
To understand how a regression kink design, or RKD, works, first consider a question that has important policy implications. “Let’s say you are interested in unemployment insurance,” Pei says. “How would increasing people’s payments impact how long they stay unemployed? This is your causal question.
“Our eyes are always playing tricks on us. The questions we’re asking, as researchers, are how do we best present data and how much can we trust our own perception?”
“Now imagine a graph where your horizontal axis contains the amount of money these people made before they lost their jobs, and your vertical axis contains the amount of money people receive from unemployment insurance during a given time,” Pei says. When these data points are plotted, the line drawn through them exhibits a kink, or sharp change in slope. That’s the result of a policy decision. To the left of the kink point, unemployment benefits go up with pre-layoff earnings. But there’s a limit to how much unemployment insurance will pay out. To the right of the kink point, the relationship is flat—people’s unemployment benefits stay the same level, regardless of what they earned before.
“Now imagine a second graph where your horizontal axis still contains pre-layoff earnings, but your vertical axis contains measures of unemployment duration,” Pei says. “It turns out that the ratio of the kink in the second graph compared to the kink in the first graph gets us the answer to our causal question.” In a study using data from the State of Missouri, Pei and another set of collaborators, which also included Card, found that during the Great Recession a one percent increase in unemployment insurance lengthened unemployment durations by about 0.8 percent.
“That’s pretty important information to have,” Pei says, “because there has always been a question in this country about the right level of unemployment benefits. If you make them too high, there is a disincentive for people to go out and get work. If they are too low, the program won’t serve its function very well as a social safety net.”
Eye-conometrics
“So much rests on our eyes,” Pei says. He attributes much of RKD’s appeal to its dramatic visual appearance. The same appeal can be found in a regression discontinuity design, a closely related method in which an economist looks for a jump, rather than a kink, in a scatterplot. “And this got me thinking, how good are we at using our eyes to evaluate evidence? We present these graphs all the time, because we think that a reader will have a reasonable ability to interpret them. But how do we know, really, they’re picking up the right things? These questions have sparked a whole new direction in empirical research for me.”
Pei’s visual inference study (“I like to call it my eyeball econometrics study or eye-conometrics,” he says) measures how accurately participants—in this case, Cornell students and experienced social scientists—identify discontinuities and kinks in graphs. “We’re using actual people in this study,” Pei says. “This is a bit new to me, and I’m pretty happy about it. Our eyes are always playing tricks on us. The questions we’re asking, as researchers, are how do we best present data and how much can we trust our own perception?”
Study participants are randomly assigned to different groups, or treatment arms. Each group is then presented with graphs constructed in a particular way. “The graphs all come from the same underlying data sets, but they present the information differently,” Pei says. “We’re trying to determine what graphs tend to elicit the most accurate responses.”
A second facet of the study compares econometricians’ visual inference with econometric software tools commonly used in the field. “We find the human eye performs quite competitively with the software when the graphs are constructed ‘right,’” Pei says.
The research platform for this study, Pei explains, is very Cornell-based. “Almost all of my collaborators have ties to Cornell, including Christina Korting, PhD ’20, and Yi Shen, PhD ’13. We did all of our randomized experiments through the Business Simulation Lab in the Johnson School, and we received funding from what is now the Cornell Center for Social Sciences. I’m very grateful we have the resources here to do this, and that it’s allowing me to keep chasing the rainbow with this study.”
For the Good and Welfare
“Initially, I was drawn to this work by the rigor of the math,” Pei says. “But, at the end of the day, I also want to be doing something that can be of value to people. My policy work fulfills this need for me, because I think these programs affect people’s lives.”
In a study currently underway, Pei and a team of collaborators are analyzing policies in the United States’ cash welfare program. The project has received more than $1 million in funding. “It’s been more than 25 years since the U.S. welfare reforms of 1996 were introduced,” he says. “Despite how much time has passed, we still know very little about the impact of welfare policies on the long-term wellbeing of recipients and their children. Our project will fill this gap.”